Like almost no other mobile service, text messaging has been one of the mobile industry’s biggest success stories. In a world of multiple devices, operating systems, and service providers, text messaging remains the one channel through which all mobile users can reliably communicate. Syniverse has had the fortune to play a key role in this technology’s development over the last 25-plus years, and even as new technologies and competitors have emerged, text messaging has continued to stay strong.
However, the durability of messaging has led it to become a prime target of fraudulent activity and suffer a soaring increase in messaging spam in the past few years. A particular problem is that since most messaging is legitimate, identifying and filtering spam while ensuring delivery of the huge volume of legitimate traffic has been a complex challenge.
Syniverse has been right in the thick of tackling this problem, and as part of this ongoing effort, I recently had the opportunity to participate in a webinar that examined the opportunity for artificial intelligence (AI) in combating messaging spam. The webinar, “The role of machine learning in enterprise communications,” also included Surash Patel, General Manager, Messaging, and Vice President, RealNetworks, as a speaker, and was moderated by Tim Green, Features Editor, MEF.
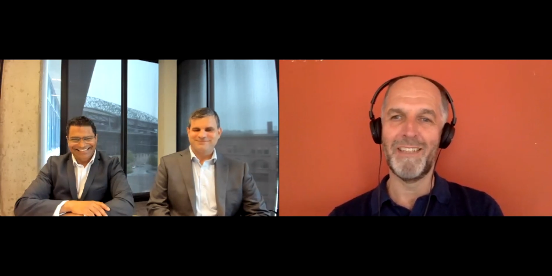
“The role of machine learning in enterprise communications” webinar.
We had a fascinating discussion that I invite you to check out in full here or get a summary of in the main points broken out from an MEF recap below. Syniverse has been right at the forefront of helping businesses make the most of text messaging, and as we progress, we’ll continue to share our latest insights here on our blog. I hope you find them useful, and I would love to get your thoughts on them in a comment below.
Filters and firewalls are ‘reactive’ forms of defense
“From a technological perspective, there’s been a lot of work around filters and firewalls,” says Surash Patel. “They look at the communications layer, at the IP address, and then analyze messages from that perspective. What we find is that these things are quite reactive. They spot things after they have happened and reprogram accordingly.”
Chris Galdun adds: “I agree it’s very static. Filters look for the IP address and for keywords after the fact and then block them. And what we find is that this is very limited because fraudsters make changes too. They will change the content of the message and the sender ID.”
Natural language processing offers a new way to analyze vast data sets
“We’re creating so much data,” says Patel. “As messaging gets so complicated we need another way of analyzing the data to make some sense of it. NLP (neuro linguistic programming) lets you understand it and see trends and relationships in it.”
“Take the example of banking notifications. They all cluster together. So a customer can look at the route these messages have taken and say ‘OK these are the legitimate senders, and these are the ones I don’t recognize or don’t think are coming from those sources’. The system is very good at solving those problems quickly.”
Galdun agrees. “Historically, on our platform we looked for spam. But spam is hard to define,” he says. “Is it true spam or just unwanted messaging? In the traditional model, this was black or white. Now, we can set up the rules differently. We can tune these rules as granularly as need be.”
But an AI system is only as good as its data set
“You look for generalizations in the data,” says Patel. “But you have to start with unbiased data. If your data is skewed, it will come to a particular conclusion. In machine learning, there’s a trade-off between ‘recall’ (how many correct interventions a system makes) and ‘precision’ (how few incorrect interventions it makes).
”We always have to balance recall with precision. We have to ask: are there instances we haven’t seen before, and did we detect them? Different industries have different priorities. We’re looking at both. We tune the model to what a particular customer needs.”
In the messaging space, an AI system can inspect traffic at the character level
“We look for messages that are very similar, and then try to understand what type of traffic it is,” says Patel. “Is it a two factor authentication? Is it a delivery alert or an appointment reminder? We have human labelers that help with this.
“But the training doesn’t stop there. We can also look at the characters within the words. For example, does it say win or w1n? Then we look at the words in a sentence and on top of that at the meta data such as: where did the message come from? Where is it going? What kind of responses is it getting?
“Finally, you train the model with validation data to see how good your model is. Then when it goes live you check for false positives and negatives to find out: did we miss something? The idea is the system gets smarter and smarter as you go.”
Galdun gives an example of how this works. “We had fraudulent traffic where the content included a URL,” he says. “That would normally indicate spam, but this happened to be the MNO’s own URL, so it got through. But because we had meta data, we could block it – or at least check with the MNO.”
If you can classify traffic, you can prioritize it
“When there is visibility into the type of traffic going over the network, MNOs can decide which is more valuable to the sender than others,” says Patel. “Then they have the opportunity to charge different traffic at different rates… or at least manage resources more effectively.
AI tools can help MNOs stay on the right side of regulation
“There’s far less human interaction with these systems – that’s a key element for any regulator,” says Galdun. There’s no human who is opening and inspecting the data. There’s also the anonymization of data. You can identify the cluster but you don’t see the PII behind the message.”